Machine learning (ML) is a subset of Artificial Intelligence (AI) that focuses on developing algorithms and models that allow computers to learn from data and make predictions or decisions without being explicitly programmed. It enables computers to automatically learn and improve from experience. Machine learning doesn’t use explicit rules like traditional programming. Instead, it learns patterns from data, enabling algorithms to make predictions and take actions based on those patterns.
History
The concept of machine learning emerged in the 1950s and 1960s as part of the broader field of artificial intelligence (AI). In the 1970s and 1980s, researchers focused on knowledge-based systems and expert systems. The 1990s saw the rise of statistical learning techniques, such as Support Vector Machines (SVM) and decision trees.
Neural networks experienced a resurgence in the 2000s, fueled by computational advancements and the emergence of deep learning. The 2010s witnessed a boom in machine learning due to big data availability, leading to breakthroughs in areas like computer vision and natural language processing. Currently, machine learning continues to advance with a focus on interpretability, fairness, and the integration of new technologies like reinforcement learning and quantum computing.
Types
Machine learning can be broadly categorized into three main types: supervised learning, unsupervised learning, and reinforcement learning.
Supervised learning
Supervised learning involves training machine learning models using labelled data, where the desired output is known. The models learn patterns and relationships from this labelled data to make predictions or classify new, unseen data. It is widely used in tasks such as image recognition, sentiment analysis, and fraud detection.
Unsupervised learning
Unsupervised learning deals with unlabeled data, where the model learns patterns, structures, or relationships without explicit guidance. Clustering algorithms group similar data points together, while dimensionality reduction techniques simplify complex datasets. Unsupervised learning finds applications in market segmentation, anomaly detection, and recommendation systems.
Reinforcement learning
Reinforcement learning involves an agent that interacts with an environment, learning to make decisions and take actions to maximize rewards. Through trial and error, the agent learns optimal strategies by receiving feedback in the form of rewards or penalties. Reinforcement learning has shown success in game-playing, robotics, and autonomous systems.
Machine learning algorithms
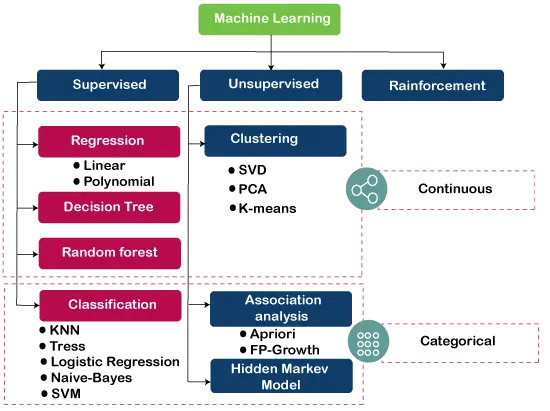
Machine learning algorithms are the core components of machine learning systems that enable computers to learn from data and make predictions or decisions. These algorithms are designed to capture patterns, relationships, and insights from the data. There are various types of machine learning algorithms, each suited for different tasks and data characteristics.
For example, supervised learning algorithms like linear regression, decision trees, and support vector machines learn from labelled data to make predictions. Unsupervised learning algorithms such as clustering and dimensionality reduction techniques discover patterns and structures in unlabeled data.
Deep learning algorithms, based on neural networks, excel at processing complex data like images and text. Reinforcement learning algorithms optimize decision-making through interactions with an environment. The choice of algorithm depends on the problem at hand, the available data, and the desired outcome.
Applications
Machine learning has found applications across a wide range of industries and domains, revolutionizing how tasks are performed and insights are generated. In healthcare, machine learning aids in disease diagnosis, personalized medicine, and medical imaging analysis. In finance, it is used for fraud detection, risk assessment, and algorithmic trading.
E-commerce platforms leverage machine learning for personalized recommendations, customer segmentation, and demand forecasting. Natural language processing enables chatbots, sentiment analysis, and language translation.
Machine learning is also applied in autonomous vehicles, predictive maintenance, energy optimization, and environmental monitoring. With its ability to analyze vast amounts of data and uncover valuable patterns, machine learning continues to reshape industries and drive innovation in diverse fields.
Challenges and Ethical Considerations
Machine learning faces challenges and ethical concerns. Bias in data and algorithms can lead to unfair outcomes. Protecting privacy and data is crucial. Understanding how models make decisions is difficult. The impact on jobs and society must be considered. Addressing these challenges is important for responsible machine learning.
Future trends
The future of machine learning holds exciting possibilities. One prominent trend is the advancement of explainable AI, aiming to make machine learning models more transparent and interpretable. Another area of focus is the development of reinforcement learning techniques, enabling machines to learn and make decisions in complex environments. Federated learning, which allows models to be trained on decentralized data while maintaining privacy, is gaining attention.
Additionally, the integration of machine learning with edge computing enables faster and more efficient inference on devices. Continued exploration of novel architectures and algorithms, as well as ethical considerations, will shape the future of machine learning, paving the way for new applications and advancements in various industries.
Achievements
There have been numerous significant achievements in the field of machine learning. Here are a few notable ones:
Image Recognition
The development of convolutional neural networks (CNNs) and deep learning has revolutionized image recognition. In 2012, AlexNet, a deep CNN, won the ImageNet Large-Scale Visual Recognition Challenge, dramatically advancing the accuracy of image classification.
Natural Language Processing
The field of natural language processing (NLP) has seen remarkable progress. In 2018, models like OpenAI’s GPT-2 demonstrated impressive language generation capabilities, generating coherent and contextually relevant text.
Autonomous Vehicles
Machine learning has played a vital role in the development of autonomous vehicles. Companies like Tesla have made significant strides in using deep learning algorithms to process visual data from cameras and sensors, enabling self-driving capabilities.
Healthcare Breakthroughs
Machine learning has contributed to significant advancements in healthcare. For example, machine learning models have been used to improve disease diagnosis, predict patient outcomes, and assist in personalized medicine.
Game Playing
Machine learning has achieved remarkable success in gaming. Notably, AlphaGo, developed by DeepMind, defeated world champion Go players, demonstrating the power of reinforcement learning algorithms in mastering complex games.
Recommendation Systems
Machine learning has greatly influenced the development of recommendation systems, driving personalized content and product recommendations on platforms like Netflix, Amazon, and Spotify.
If you enjoy reading our articles, take a minute to subscribe to our Newsletter. Click Here! (It’s Free, No Spam!)
Additional Reference -> Click Here!